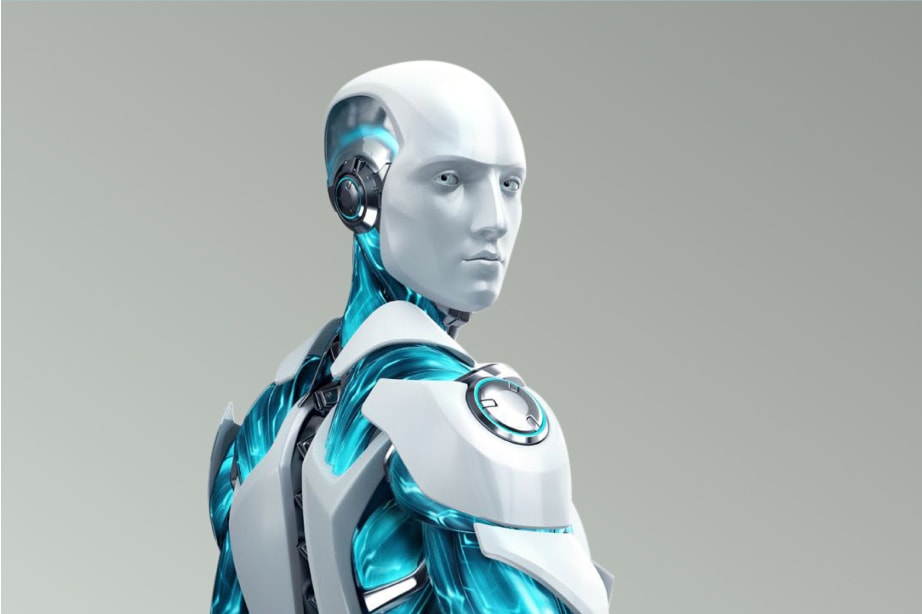
VTC Academy | AI Practitioner
AI Practitioner
Course format: On campus
Duration: 8 weeks
Major: Artificial Intelligence
ENROLL NOWIntroduction
The AI Practitioner Course provides an overview of AI relating to introduction to AI/Machine Learning, Common Machine Learning Algorithms, AI Applications.
Especially, the course helps students approach AI through the TEFPA framework (Task, Experience, Function space, Performance measure, Algorithm to search/optimize) – an exclusive Machine Learning approach framework developed by VTC Academy and COTAI.
Applicable areas of course
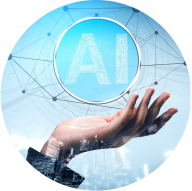
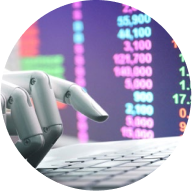
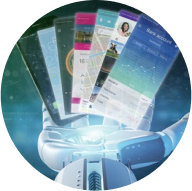
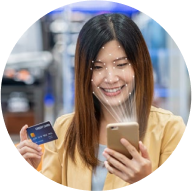
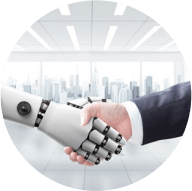
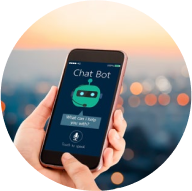
Who can learn?
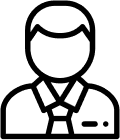
GROUP 1: People who do not have advanced Math and Python programming background * (Need to take Pre-Practitioner class (4 weeks) before starting)
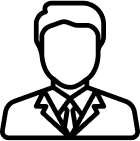
GROUP 2: People with advanced Math and Python programming background *
* Subject to the International-standards-based competency assessment of VTC Academy
Course overview
- Introduction to AI/Machine Learning, common machine learning and AI practice methods
- TEFPA framework (Task, Experience, Function space, Performance measure, Algorithm to search/optimize), an exclusive Machine Learning access framework developed by VTC Academy and COTAI
- Math/Computer/Programming/Data
- Machine Learning and Deep Learning Models
- Visual & Language Understanding, Decision Making with pre-trained Deep Learning models
- Step-by-step development of AI applications
Course outcomes
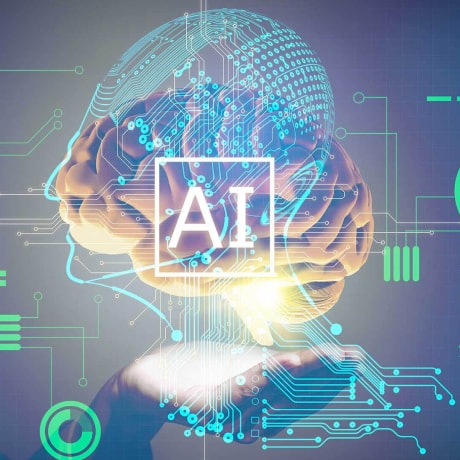
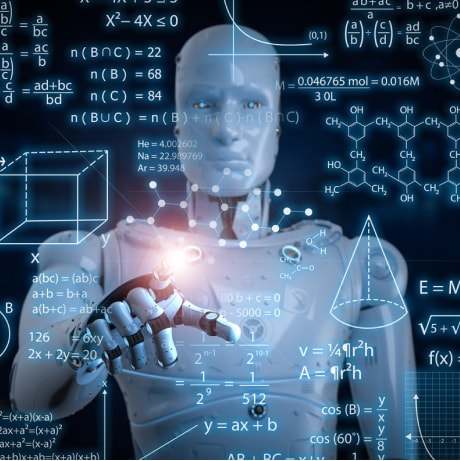